Creating value from data: How AI agents conquered the year 2024
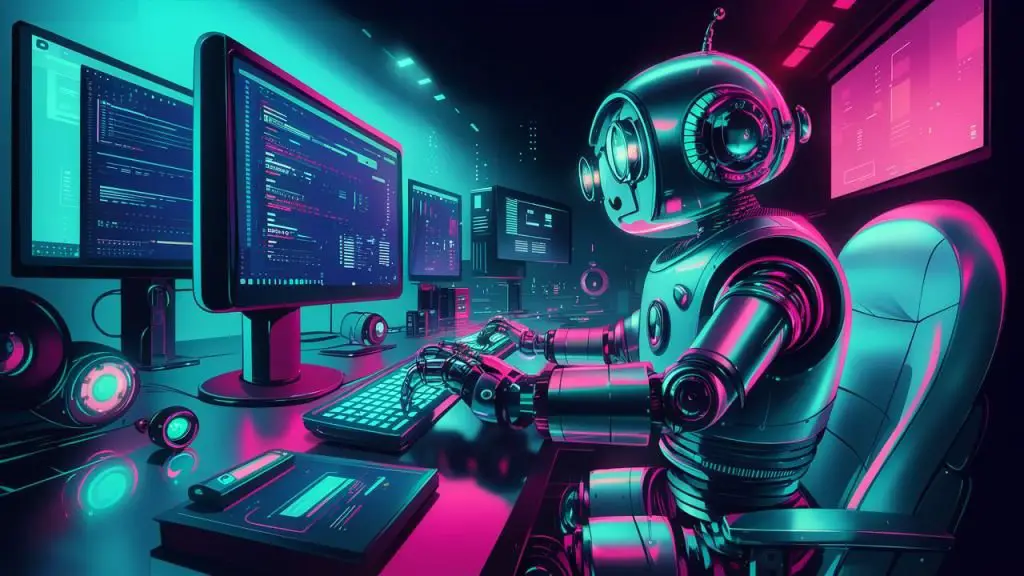
Subscribe to our daily and weekly newsletters to receive the latest updates and exclusive content on industry-leading AI reporting. Learn more
If 2023 was the year of generative AI-powered chatbots and search, The year 2024 was all about AI agents. What started at Devin earlier this year grew into a full-blown phenomenon, offering companies and individuals the opportunity to transform the way they work on various levels, from programming and development to personal tasks like planning and booking tickets for a vacation .
Among these far-reaching applications, we also saw the rise of Data agents this year – AI-powered agents that perform different types of tasks across the data infrastructure stack. Some did basic data integration work, while others handled downstream tasks like analysis and management in the pipeline, making the job simpler and easier for enterprise users.
The benefits included improved efficiency and cost savings, leading many to ask: How will things change for data teams in the coming years?
Gen AI agents took over data tasks
While agent capabilities that allow companies to automate certain basic tasks have been around for some time, agent capability development is on the rise generative AI has completely taken things to the next level.
With Gen AI’s natural language processing and tool usage capabilities, agents can go beyond simple thinking and responding to actually plan multi-step actions. They can independently interact with digital systems to complete actions while collaborating with other agents and people. They also learn to improve their performance over time.
Devin from Cognition AI was the first major agent offering to enable technical operations on a large scale. Then larger providers began offering more targeted corporate and private agents based on their models.
Speaking to VentureBeat earlier this year, Google Cloud’s Gerrit Kazmaier said he’s heard from customers that their data professionals are constantly facing challenges, including automating manual work for data teams, reducing the cycle time of data pipelines and analytics, and simplifying of data management. Essentially, teams didn’t lack ideas about how to create value from their data, but they lacked the time to implement those ideas.
To address this issue, Kazmaier explained, Google revamped BigQuery, its core data infrastructure offering, with Gemini AI. The resulting agent capabilities not only provide organizations with the ability to discover, cleanse, and prepare data for downstream applications—breaking down data silos and ensuring quality and consistency—but also support pipeline management and analysis, freeing teams to focus on higher-value tasks can.
Today, many companies use Gemini’s agent capabilities in BigQuery, including fintech companies More thanwhich leveraged Gemini’s ability to understand complex data structures to automate its query generation process. Japanese IT company Tireless also leverages Gemini’s SQL generation capabilities in BigQuery to help its data teams deliver insights faster.
But discovering, preparing and supporting the analysis was just the beginning. As the underlying models evolved, even granular data operations – developed by startups specializing in their respective domains – were targeted with deeper agent-driven automation.
For example, AirByte And fast made headlines in the data integration category. The former launched a wizard that created data connectors from an API documentation link in seconds. Meanwhile, the latter expanded its broader application development offering to include agents that generated enterprise-class APIs – be it for reading or writing information on any topic – using only a natural language description.
Based in San Francisco Altimate AIfor its part, targeted various data operations, including documentation, testing and transformations, with a new DataMates technology that leveraged agentic AI to get context from the entire data stack. Several other startups including Red bird And RapidCanvasalso worked in the same vein, claiming to offer AI agents that can handle up to 90% of data tasks required in AI and analytics pipelines.
Agents supporting RAG and more
Beyond broad data operations, agent capabilities have also been explored in areas such as retrieval-augmented generation (RAG) and downstream workflow automation. For example, the team behind the vector database Weave I recently discussed the idea Agent RAGa process that allows AI agents to access a wide range of tools – such as web search, calculators or a software API (like Slack/Gmail/CRM) – to retrieve and validate data from multiple sources to ensure the accuracy of the improve answers.
Furthermore, towards the end of the year, Snowflake intelligence appeared, giving companies the ability to set up data agents that could access not only business intelligence data stored in their Snowflake instance, but also structured and unstructured data through isolated third-party tools – like sales transactions in a database or documents in knowledge bases like SharePoint and information in productivity tools like Slack, Salesforce and Google Workspace.
With this additional context, agents deliver relevant insights in response to natural language questions and take specific actions around the insights generated. For example, a user could ask their data agent to enter the displayed insights into an editable form and upload the file to their Google Drive. You could even be asked to write to Snowflake tables and make data changes as needed.
There is much more to come
While we may not have covered every data agent application seen or announced this year, one thing is pretty clear: the technology is here to stay. As generational AI models continue to evolve, the adoption of AI agents will continue at a rapid pace, with most companies, regardless of their industry or size, opting to delegate repetitive tasks to specialized agents. This will be directly reflected in increased efficiency.
Proof of this is a recent survey of 1,100 tech executives conducted by Capgemini82% of respondents said they plan to integrate AI-based agents into their stacks within the next three years, up from 10% currently. More importantly, 70 to 75% of respondents said they would trust an AI agent to analyze and synthesize data on their behalf, as well as perform tasks such as generating and iteratively improving code.
This agent-driven shift would also mean significant changes to how data teams function. Currently, the agents’ output is not production-ready, meaning that at some point a human will need to take over to adapt the work to their needs. However, with some further advances in the coming years, this gap will most likely be closed and teams will get AI agents that are faster, more accurate and less prone to mistakes usually made by humans.
In summary, the roles of data scientists and analysts we see today are likely to change, with users potentially moving into the realm of AI monitoring (where they could keep an eye on the AI’s actions) or higher-level tasks as Changing the system may have difficulty performing.
Source link